I just returned from an event in Hoboken where PhuSE brought organizations together to explore a hot topic that's being discussed in our industry: Artificial Intelligence (AI) and the Transformation of Healthcare.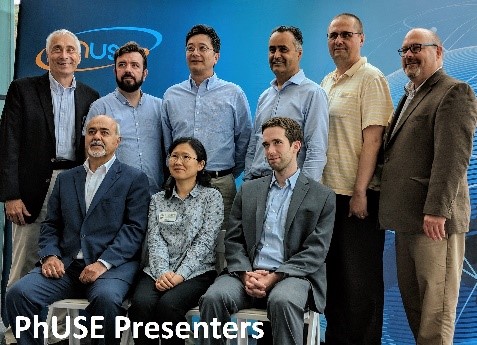
A number of presenters from academia, health care, and consulting and pharmaceutical/biomedical companies described their innovative implementations of AI to unlock data and answers in life science settings through analysis of Real World Data (RWD) and Real World Evidence (RWE). d-Wise follows such cutting edge technology vigilantly as this is directly in line with our mission is to create innovative solutions that connect, accelerate, and customize enterprise technologies within life sciences development. Our key takeaways from these presentations were the following:
The applications and data sources for AI technology in our life science industry are many and far ranging. Among the applications described were:
- Automating literature review based on selected journal articles
- Matching patients to clinical trials based on the ClinTrials.gov database
- Development and use of a predictive model for disease diagnosis based on Electronic Health Record (EHR)
- Classification of patient subgroups/phenotypes based on coupling claims data with EHR
The methodologies underpinning the use of Machine Learning (ML) and in applicable cases Natural Language Processing (NLP) are similar across most of these applications. An area of particular focus for all presenters was in exploring the refinement of those methodologies for faster and more accurate results. One presenter explained how the Julia language, which is capable of performing ML and can run Python and R, has processing speeds far faster than Python, R, or SAS.
Also common across the applications described were the following challenges:
- Identifying and gaining access to the right data to use
- The availability of linked databases
- Understanding the data – clinical meaning, structure, assumptions, relationships, etc.
- Overfitting of the data due to small sample size
- A need to incorporate greater context of isolated pieces of data
All presenters agreed that implementing AI technology within a company will widen even more the divide between those with specialized technical expertise and those with business/clinical knowledge. The key to success will be learning to understand each other. Regardless of which side your skills lean, attending presentations like these will go far to enhance your understanding of the other side. I'm looking forward to the next PhUSE event!